With mobile technology accessibility expanding worldwide, new strategies to collect data have emerged. Humans can now act as sensors through internalizing external physical or social phenomena via their mobile technology. For example, as a smartphone owner, I can remotely take a photo of an experience, say a sunset, and then I can publicize this experience through uploading the photo to a social media platform or website. Apart from the photo, I can also add additional information, such as a text description or geotag. In this case, the product, the publicly shared geotagged photo with a description, is volunteered geographic information (VGI), which is any user-generated content that is spatially referenced. My contribution is both explicitly georeferenced through the geotag, but it is also implicitly georeferenced through the description. VGI has been used in several projects as a mechanism to collect and analyze geographic information. This approach has created a new model for collecting geographic information that diverges from the traditional model, which relies on expert cartographers travelling to the field, surveying the land, and recording spatially-referenced attributes.
For example, the Commission for Environmental Cooperation (CEC) has developed the Local Environmental Observer (LEO) Network. This crowdsourcing project was developed to collect reports on unusual environmental phenomena in North America. Rather than hiring individuals to monitor, the LEO Network uses crowdsourcing as a mechanism to monitor the environment. Reports are recorded through a user placing a point on a map and adding specific information detailing the phenomenon. This allows the contributor to provide both explicit and implicit geospatial information. In this case the users are actively engaging in the project, but this is not necessarily the case for all projects that use VGI to collect geographic information. Rather some focus on automated, algorithmic, approaches to collect geographic information from individuals.
In a sense, there seems to be a separation in VGI research between machine learning and collaborative learning; in other words: unconscious versus conscious volunteered spatial data. The latter focuses on encouraging individuals to consciously volunteer their geographic data to a public forum. For instance, Humanitarian OpenStreetMap (HOT) has created a platform for OpenStreetMap (OSM) users to actively contribute to tasks necessary to complete missing maps for projects. Organizations who collaborate with HOT, such as MissingMaps, have come up with open source tools that make contributing easy, through seamless UI and gamification. Actively engaging participants can be valuable because local knowledge is considered and locals can feel empowered for contributing; moreover, conscious VGI can provide a more collaborative environment that encourages enthusiasts. However, these sorts of VGI projects can lack sustainable contributions; for instance; people lose interest over time when they are not fiscally compensated for their contributions.
As an alternative, automated methods via algorithms have become a new solution to offset low levels of active contributions. Automated processes will certainly benefit Smart Cities; for example, Google crowdsources Android GPS data to provide traffic reports, which over time could be integrated in self-driving cars to determine which routes to take to avoid traffic. In another case, machine learning can be used to detect buildings in satellite imagery. Automated methods will become extremely useful for real-time sensitive realities, such as a natural disasters.
Although there are benefits to automated approaches, there are critiques, particularly on validity because it is difficult to know whether the data is correct when contributors are not actively engaged. This can cause systematic errors in the long run. For example, a team can create an algorithm to mine and analyze Tweets that are spatially referenced. This team considered spatially referenced Tweets to be ones that are either geotagged or ones in which the user has a registered location, in other words, the user’s profile location. However, since you can geotag a tweet to a location that is not your current one, it is difficult to confirm whether a geotagged post is in the same location as where the user sent the post; moreover, just because a user’s account has an assigned location, does not mean they are actively there. I myself, have mined Tweets and spent several hours staring at my datasets overwhelmed with spatial uncertainty.
The debate between the advantages and disadvantages of machine and collaborative methodologies to harnessing VGI is emerging and growing, with recent literature even questioning whether volunteer is misleading. Whether or not one approach is the ultimate solution is not up for debate, rather I believe we need to recognize the importance of incorporating both in VGI projects because, as stressed above, VGI projects incorporate humans, technology, and geographic space. Consider Google Maps, although they focused most of their time using automated methods and their employees to create Maps, they are now expanding their platform to make it more suitable for crowdsourcing (similar to OSM). Although Google still is not opening up their geospatial data, they are attempting to account for local knowledge. They recently have started a project that encourages Google Map users to report accessibility for handicap individuals, this way Google Maps will consider accessibility for handicap individuals when routing directions.
- Julia Conzon
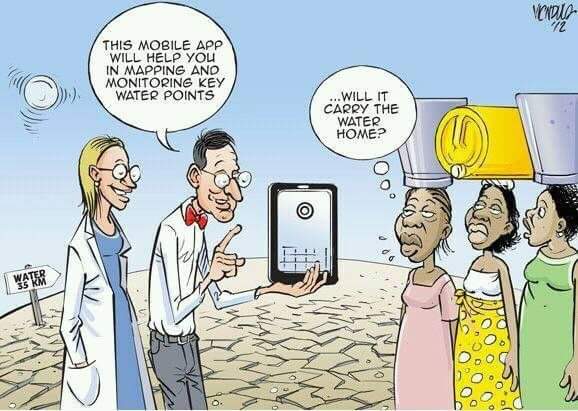